Why Culture and Architecture Matter with Data, Part I


We are using data wrong.
In today’s data-driven world, we have learned to store data. Our data storage capabilities have grown exponentially over the decades, and everyone can now store petabytes of data. Let’s all collectively pat ourselves on the back. We have won the war on storing data!
Congratulations!
Does it really feel like we have won, though? Or does it feel like we are losing because the goal was never to store data? The goal was to use our data, hopefully at increasing speeds, for such use cases as product improvement, increased profits, lower costs, increased customer satisfaction, user experience, lower downtime, security, and so much more. Now most of us realize that innovation costs are the actual consequence of not leveraging our data.
Studies and surveys paint a grim picture of current enterprise efforts related to data. According to Harvard Business Review, "Cross-industry studies show that on average, less than half of an organization's structured data is actively used in making decisions—and less than 1% of its unstructured data is analyzed or used at all."
Put simply, we want to use the data for our benefit but have not yet figured out how. Once data is stored, it might as well fall into a black hole, never to be seen again. To this point, the promises of Big Data have gone unfulfilled.
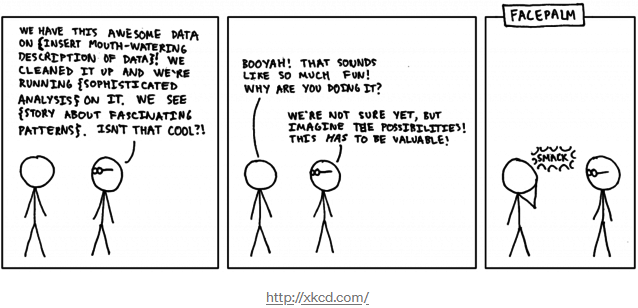
Does this deter companies from continuing to invest in data initiatives, though? Not according to 91% of those surveyed by NewVantage Partners, where businesses reported they were increasing the amount of money spent on data initiatives. This continued commitment has been a growing trend for over a decade.
Doing the Same Thing Over and Over Again, Expecting a Different Result
In the face of so much failure, why are companies dedicating significant time, resources, and people to chasing what feels like data insanity? A straightforward answer is that the precedent was set by a small group of companies using data correctly. They were the first movers who learned the lessons first. These companies and their stories from afar can feel like magic to the companies that have yet to accomplish the same success. The stories of how successful companies leveraged their data are the stuff of legend and can both inspire while also creating fear of being left behind.
For example, one company in the entertainment space put its largest competitor out of business while simultaneously building a new business model. It harnessed the power of its data by becoming data-driven while enabling its employees, through a strong data culture, to create a data architecture and tooling to find critical insights and make important business decisions in a fraction of the time of their competitors.
Another company in the retail space adopted a data-driven approach, and a modern cloud architecture, to burst needed computing resources into their cloud provider during the busy holiday season, saving tens of millions of dollars annually.
Data-driven companies innovate faster, and losing out on innovation to your competition is the death knell for a business. Therefore billions of dollars spent annually in the enterprise on data platforms, architectures, and people is not insanity but the requirement to evolve companies into modern businesses. It’s the cost needed to pay for innovation.
Enterprise Data Challenges
Companies are putting up the capital and have the desire to become data-driven. So what are examples of the challenges that prevent them from using their mountain of data?
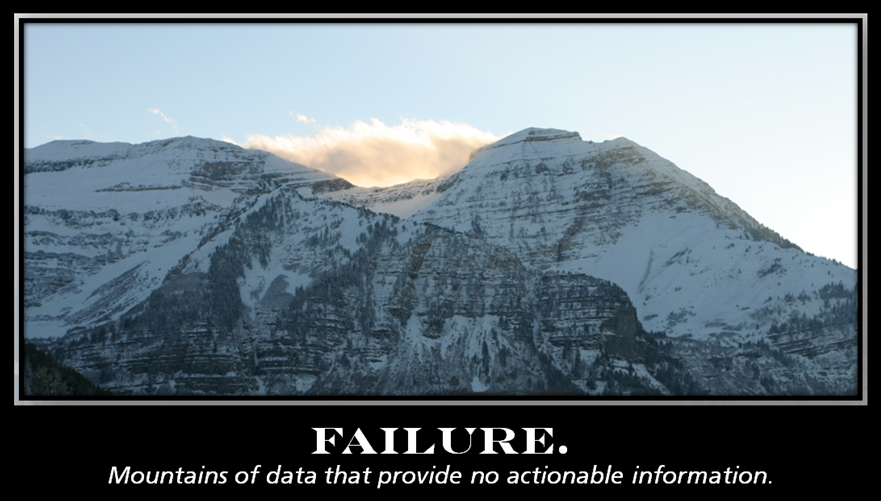
The first challenge to call out is the traditional data architecture which primarily focuses on moving data horizontally from source to destination with a data pipeline. These architectures make transporting and storing data more efficient but do little to extract business value from the data. A longstanding problem with this architecture is siloed ownership of the data at the destination to the teams that create and primarily use the data when the goal should be to make it easy to access for any business use case and internal team. While this architecture was suitable for transporting increasing data volumes and a necessary step in the data architecture evolution, it did little to address the complexities for internal teams to work with data or their desire to pull data from across data domains.
Next up, closely associated with the first, is how data in the enterprise is treated more as an object than a business asset. In the last fifteen years, we've created modern, highly distributed cloud architectures that horizontally scale to load and have forever changed engineering team design, cultures, and operations to support them. And yet, for the last sixty years, we have continued to store data in legacy monoliths. Telemetry, customer, marketing, and sales data go into separate silos. This separation of the data prevents finding valuable business insights into how a decision made in one part of the company affects another. This siloed data, as the foundation of the business data domains, prevents or hinders the company from using valuable correlation across those domains to glean critical insights to propel the business.
Next is how to effectively enable the people responsible for the engineering lifecycle of data from data creation to consumption. The concept of data engineering isn’t new but has become challenging based on modern cloud architectures, data volume increases, and the different expectations created for the data. In the everyday engineering world, trying to work with data at a cloud scale is arguably known by few. Just working with data is hard enough without throwing in the myriad of technologies underlying the pipeline architecture. Data, pipeline, and technologies problems mixed in with the necessary skill set and complex troubleshooting tasks are a tall order for any one person to be responsible. And I didn’t even mention security, compliance, and data governance… the scary bogeymen in the room that can paralyze decision-making for data use cases.
There are also the challenges of team scale. The larger the enterprise, the higher the need for technical specialization in one or more of the expertise needed to work with data. And this leads to entire teams who uniquely understand their piece in the pipeline ecosystem while being frustrated by their lack of awareness of the problems related to data elsewhere in their team. When issues arise with the pipeline/data/correlation, who owns which piece? At a smaller enterprise scale, without all the specialized groups, it is hard to hire people who understand all the challenges and have the necessary skills to work with data. Whether the team is large or small, there are unique challenges with either.
Above all of these challenges, there is the fact that people, data, and technology alone do not ensure better decisions unless one component revolves around supporting them: the data-driven culture.
Every company desires to be more data-driven, but a new approach to data, pipeline architectures, and the people operating inside them need help and support. In recent memory, a cultural shift was necessary with the DevOps & SRE movement to implement changes to support distributed architectures. Likewise, companies need a culture shift to achieve the success they desire with their data.
While these challenges can feel daunting to the teams and individuals who live them daily, there is hope. Primarily coming from the companies and even individuals who have addressed data problems with new patterns, architectures, and norms which are forming to raise the expectations of what it means to work with data. In my next article, I'll go over what is happening with the customer, technology, and startup world to address the challenges of working with data.
SHARE ARTICLE
RELATED ARTICLES